Leading vs. lagging indicators: What’s the difference?
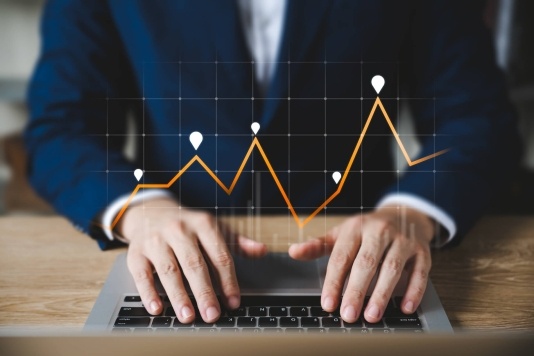
To understand the present, we must know the past. To create the future, we have to take action in the present. This fundamental law of the universe speaks to an important data analysis concept when looking at the future potential of your organization: Leading versus lagging indicators. If we want to understand who we are, we use our memory to reflect on the events that shaped us. When we plan for our future, we use that knowledge to search for signs of what’s to come. We may not have a crystal ball, but we can recognize repeated patterns that have led to desirable or undesirable outcomes.
While organizations don’t share our gifts of memory or pattern recognition, they have lots of data. By organizing data into properly defined “lagging” and “leading” indicators, organizations improve their ability to predict future outcomes. The only way for organizations to perceive and understand leading and lagging indicators is through effective quantitative and qualitative research methods.
What is a leading indicator?
Leading indicators signify possible future conditions in a market or business. They’re heavily used by economists, researchers, investors, risk managers, and more to forecast the future.
To establish leading indicators, a researcher’s perspective shifts to being future-focused. Leading indicators are action-oriented and should define actions necessary to achieve goals. They’ve established benchmarks by which an organization can form hypotheses on upcoming dips or peaks.
Related reading: How proactive qualitative research drives innovation and enriches quantitative data
Examples of leading indicators
Instead of asking what happened, leaders now ask: What might happen? What effects might this data have? What changes need to take place organizationally? What areas of improvement are most important? What initiatives need to be shut down, and what ideas should be tested? Leading indicators look at the current state of different data sets to predict what’s to come.
One of the simplest examples of leading data can be seen in economics. Economists look at leading indicators like consumer confidence, productivity, retail sales, and unemployment rates to forecast future economic health. For many organizations, finding the right leading indicators requires a proactive approach to gathering qualitative data and quantitative analytics. Often, it’s the understanding of current human emotions that best foreshadows upcoming trends.
Quantitative leading indicators | Qualitative leading indicators |
---|---|
|
|
|
|
|
|
|
|
|
|
|
|
|
|
|
What is a lagging indicator?
Lagging indicators point to the present state of an organization, measuring past production and performance. The term lagging indicator generally refers to the classic conception of data analysis and well-known performance metrics. Lagging indicators are simple to measure because they’re derived from snapshots of the past. This gives leaders insight into the current state of their organizations so that they know where they need to devote attention.
Lagging indicators’ weakness is their focus on output rather than the outcome. Organizations solely focused on output tend to cut corners that can lead to customer dissatisfaction and subpar experiences. One way to think about this is with the analogy of a school system. If teachers only teach students how to answer the exact questions on the test, it opens the door for cheating and “gaming” the system. No one learns anything. This is because the system has focused too hard on the metric of test scores and lost sight of the overall goal of a holistic, quality education. That doesn’t mean test scores are unimportant. They don’t fully capture the ultimate goal.
Examples of lagging indicators
To find lagging indicators, researchers ask questions that look into past events or timeframes. They ask questions like, How many people were involved? What was their level of engagement? What results came from that event? We’ve listed some examples of quantitative lagging indicators, in no particular order, below.
Quantitative lagging indicators | Qualitative lagging indicators |
---|---|
|
|
|
|
|
|
|
|
|
|
|
|
|
What is data exhaust?
To understand the evolution of lagging and leading indicators, it’s important to mention some important trends in today's world of data. In the digital landscape, lagging and leading indicators usually fall under “big data.” This phrase generally refers to data sets too large to be inspected record-by-record. While many people know that organizations collect this user data, most don’t realize how much data is being collected. Resting outside, on the periphery of big data, is called data exhaust.
So, what exactly does data exhaust mean? The lines between “big data” and “data exhaust” are blurred. But in the example of a bank, big data could refer to the debit and credit information in customers’ accounts. At the same time, data exhaust might be data on money withdrawal preferences, log files, bank machine information, web browser information, and anything else captured.
While big data is always attached to a core business function, data exhaust doesn’t always have a clear function. Or at least it doesn’t have a clear function yet. It’s raw and unstructured information. That doesn’t mean, however, that it’s useless. Data exhaust is important to be aware of because it represents one of the big opportunities for customer-centric organizations in the future. Just because the purpose or value of the data isn’t clear right now doesn’t mean it won’t be valuable someday. Many organizations are already saving data exhaust for later use or sale to other companies. Tech companies do this because they know that the unstoppable march of technological innovation will someday be able to leverage user data in ways we can’t even dream of right now.
Related reading: Thick data and big data: Why you need both to make user-centered decisions
Today’s data exhaust could become big data’s leading indicators of tomorrow. Because of that, the more organizations can implement reliable systems for lagging and leading indicators, the better-equipped they’ll be to use that data in the future.
Want to learn more?
Explore UX best practices, expert advice, user research templates, and more.